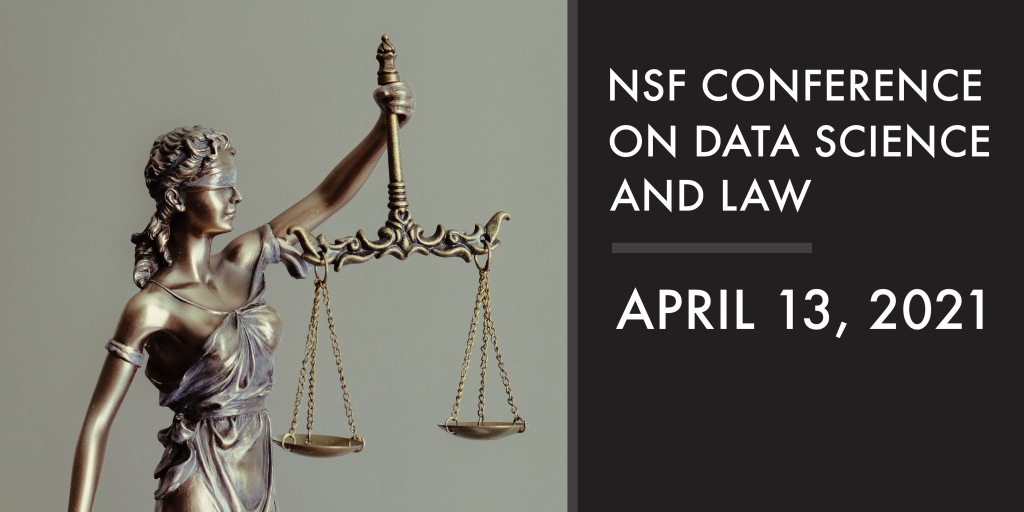
Data Science and Law are both disciplines that have perceived high barriers for entry. With data science, outsiders are overwhelmed by the thought of having to understand hard math and complicated computer code, as the Chief Justice of the Supreme Court demonstrated when he called statistical evidence of political gerrymandering “sociological gobbledygook.” With respect to law, computer and data scientists feel unequipped to interpret the fairness and justice of their work and perhaps do not even see it as relevant. Many data practitioners believe, “I am just writing an algorithm. It’s math and data; I’m not responsible for what happens downstream.”
“As data increasingly affects all aspects of daily life, we cannot continue to let data science exist in a vacuum, without thinking of the legal, ethical, and societal implications that result from that math and data. We are being reminded daily of the inadequacy of legal frameworks and lack of governmental oversight of data protection, privacy, and security,” said Sarah Davis, senior project manager at RENCI. “Similarly, legal practitioners and researchers cannot ignore or willfully misunderstand the opportunities and dangers of a data-centric society. Increasingly, ‘black box’ algorithms will be used to make decisions that may attack privacy rights, violate due process, or discriminate against protected groups.”
Davis, who worked as an attorney prior to joining RENCI’s project management team, recognized the community effort and conversation required to create bridges between data science and law. To address this challenge, Davis partnered with the National Science Foundation (NSF) and assembled a planning committee with representatives from RENCI, UNC Department Of Psychology and Neuroscience, Georgia State University, UNC School of Law, NC State University Department of Industrial and Systems Engineering, UNC Department of Public Policy, Duke University School of Law and the National Courts and Sciences Institute. The end result was the NSF Conference on Data Science & Law, an event where experts and researchers in law, data science, computer science, and the social sciences could gather virtually to exchange ideas and develop promising avenues of research to bridge the gap between the two subjects.
The event began with a welcome and a statement of the goals for the conference, which included building an interdisciplinary community of law, data science, and social science practitioners to develop a new research network; highlighting the most promising theoretical frameworks and avenues of research, both for conference attendees and for the larger interdisciplinary communities; and keeping ethical decision making at the forefront in both law and data science.
“There is an assumption among scientists from all backgrounds that it is not their responsibility to adjudicate the potential uses or ethical implications of their research. By hosting this event, we intend to build a community that will help enrich our understanding of the legal concepts and frameworks that exist in the system in order to better work within it.” said Stan Ahalt, director at RENCI. “There are substantial areas of research interest exactly at the intersection of data science and law, including artificial intelligence, cybersecurity, and health data management. For example, the NIH HEAL Initiative is focused on studying the opioid crisis, which poses several questions with significant health, data science, and law implications.”
Following introductions, the conference featured lightning talks and breakout sessions on four key topics that overlap in both fields. During the breakout sessions, attendees gathered their thoughts by using EasyRetro boards. A summary of topic abstracts and remaining research questions outlined by attendees can be viewed below.
- Data Governance Standards for Research: Nearly every organization is now a data-driven organization, and organizational leaders in every corner of society must make data governance decisions to best serve their constituents. This topic asks how can data be leveraged in research to inform decision-making in a way that protects important social needs; how can various data models serve social data governance needs; what comprehensible/accessible criteria might organizations use to make choices about data access in order to serve all stakeholder needs; and how data governance characteristics can be communicated clearly through common language and accessible decision-support tools.
- Evaluating Data Science Evidence and Experts: As Big Data becomes more widespread and central to people’s lives, the need to evaluate data properly becomes more pronounced, especially in the context of legal disputes. This topic covers issues such as the legal admissibility of data science evidence, the ability of legal factfinders to understand complex scientific evidence, and how data science experts can communicate their work effectively in court. With regards to admissibility, there is a need to establish norms for data validity, data veracity, and data provenance. Similarly, communicating the results of data analysis methods will require that the courts establish some baseline expectations on how complex quantitative information can be explained in a comprehensible manner.
- Data and Legal Frameworks in the Digital Space: The politicization of the digital space is a growing area of inquiry. However, regulation of civic engagement online is inconsistent and digital-political phenomena are inherently difficult to measure. Researchers explored the policies and laws that regulate (or do not regulate) content online and the private firms that host this content; how private firms are creating and enforcing de facto restrictions on expression and speech; and the types of, integration of, and gaps in data to study these topics.
- Developing a Taxonomy of Algorithmic Bias: Algorithms promise decision making divorced from human intervention, and therefore human bias, but this view has proven naive. In this session, we examined the sources of bias in algorithmic training and use, the role of algorithms in perpetuating pre-existing bias, and the goal of detecting and eliminating algorithmic bias. Legal experts and data scientists discussed the role of algorithms in the law, including algorithms’ impact on areas such as criminal and employment law and legal limitations on the design and use of algorithms.
Next steps for attendees include seeking additional guidance with NSF to align with its goals, conducting a careful analysis of the EasyRetro boards used during breakout sessions, and recruiting volunteers to continue the conversation and momentum until the planned fall meeting.
At the fall conference, researchers plan to continue to work towards advancing relevant theories from the natural and social sciences, lowering the barrier for entry, and creating on-going conversations that promote fairness in data science and clarity in the legal implications of data-driven decision making.
If you’re interested in learning more or getting involved with future events, please contact Sarah Davis at sdavis@renci.org.
By Sarah Davis, Senior Project Manager, and Jayasree Jaganatha, Communications & Marketing Specialist, RENCI