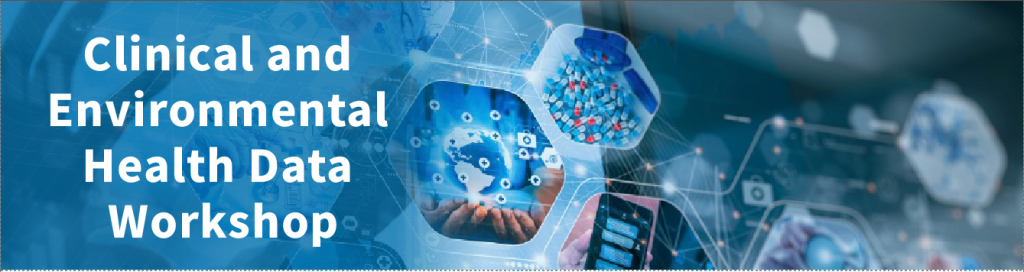
On Friday, February 23, 2024, RENCI hosted the second workshop in a series on Clinical and Environmental Health Data, themed “Integrating Exposures Data into Clinical Data Assets: Building a Regional Center of Excellence.” The inaugural workshop, themed “Clinical and Environmental Health Data Workshop Series – Exploration,” was also hosted by RENCI in May 2023.
The workshop series is being jointly led by experts in clinical and environmental health data and cyberinfrastructure at RENCI, US EPA, and NIEHS. The overall goal of the series is to leverage the wealth of expertise, resources, and organizations focused on clinical and environmental health within the RTP region and the broader State of North Carolina and establish a Regional Center of Excellence in Clinical and Environmental Health.
The second workshop built off of the success of the first workshop and the gaps and opportunities that were identified in that workshop, namely interest in exploring a regional environmental exposures data hub, the need for more timely release of environmental exposures data and models, and the need for tools to integrate environmental exposures data with electronic health record (EHR) data and EHR-like data.
The workshop convened a group of ~30 regional experts in clinical informatics, EHR data, environmental exposures modeling, environmental health, and community health, with broad representation from academic, industry, and federal organizations within the RTP region.
Two working sessions served to focus the workshop discussions and activities. The first working session, titled “Developing exposures models and releasing them in a more timely manner,” was moderated by Kyle Messier, Stadtman Tenure-Track Investigator in the Division of Translational Toxicology at NIEHS. The discussion focused on collaborative development of open-source environmental exposures models and tools and their application to real-world use cases, focusing on how environmental exposures models can better serve clinical and epidemiological studies. The second working session, titled “Applying exposures models to EHR and EHR-like data,” was jointly moderated by Emily Pfaff, Assistant Professor and co-Director of Informatics and Data Science at NC TraCS, and Cavin Ward-Caviness, Senior Computational Biologist in the Public Health and Integrated Toxicology Division at the US EPA. The discussion focused on challenges and solutions for linkage of clinical and environmental exposures data and how common clinical data models such as OMOP data might facilitate the linkage. The group also discussed a proposed NC Environmental Exposures Data Hub and related efforts such as UNC’s Enviroscan, Duke’s Seed Health Atlas, and the NC Department of Health and Human Services’ Environmental Health Data Dashboard.
One of the major outcomes of the second workshop was the development of a high-level outline for a workshop publication intended to lay out the vision and structure for the proposed Regional Center of Excellence. Workshop participants are now working toward developing a full publication and a shared vision for the center.
Workshop Planning committee: Ashok Krishnamurthy (Director of RENCI and co-Director of Informatics and Data Science at NC TraCS), Karamarie Fecho (Research Affiliate at RENCI and President of Copperline Professional Solutions), Jessica Natonick (Research Project Coordinator at RENCI), Cavin Ward-Caviness (Senior Computational Biologist at US EPA), and Charles Schmitt (Director of the Office of Data Science at NIEHS).
For those interested in learning more about the Clinical and Environmental Health Workshop Series, please contact Jessica Natonick at jnatonick@renci.org.