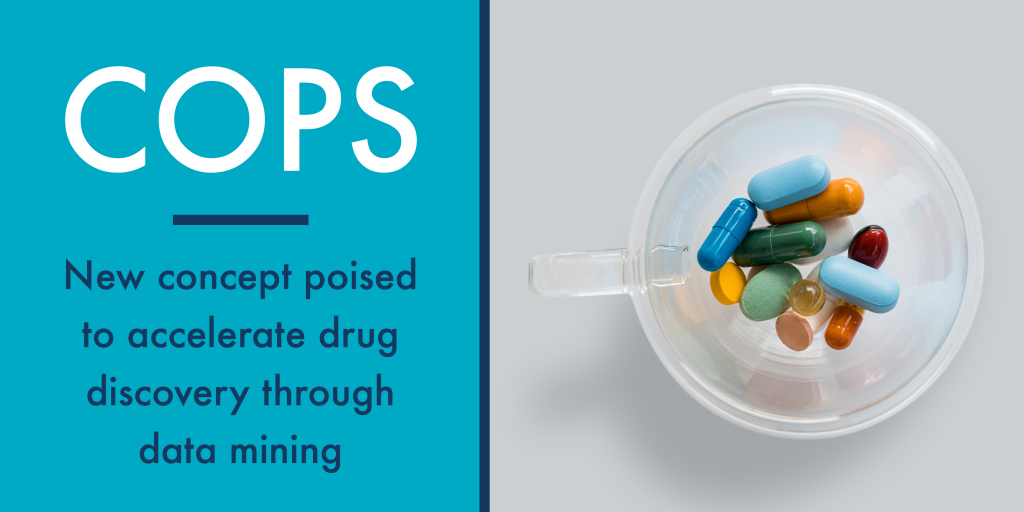
RENCI scientists together with collaborators from UNC and other institutions have developed and defined a concept called Clinical Outcome Pathways (COPs) that could help scientists harness the vast amounts of clinical and biomedical data available today to accelerate drug discovery and drug repurposing.
“Improving drug discovery requires understanding all the biological processes involved in how drugs work,” said the paper’s first author Daniel Korn from the UNC-Chapel Hill Department of Computer Science. “COPs help broaden the concept of a drug’s mechanism of action so that knowledge graph mining can be used to discover the complete chain of events that enables a specific therapeutic effect for a drug.”
Knowledge graphs express data as a collection of nodes—such as drugs and diseases—with edges that represent the relationships—such as drug A treats disease B—between the nodes. By bringing together heterogeneous information into a single system, knowledge graphs can reveal relationships between previously unconnected information that wouldn’t be obvious otherwise.
“The real power of the COPs concept is that once we understand all the biological pathways connecting drugs and diseases, that information can be used to develop new therapeutic agents—or repurpose existing ones—that modulate the same biological pathway,” explained the paper’s senior author Alexander Tropsha from the UNC Eshelman School of Pharmacy.
As described in a Drug Discovery Today paper, the researchers define COPs as a chain of key events—molecular initiating event, intermediate event(s), and the clinical outcome—that are responsible for the therapeutic actions of a drug. Each element of the chain corresponds to a term defined in commonly used biomedical ontologies, which allows computational methods to be used to elucidate COPs and provides a way for them to be cataloged for future use.
Better drug discovery
Many of today’s new drugs are designed to act on the same point in a biological pathway as existing drugs. “This creates a bunch of ‘me-too’ drugs that don’t actually increase our overall ability to cure disease,” said RENCI’s Chris Bizon, a co-author of the paper. “COPs and knowledge graphs could allow scientists to understand the full set of events involved in a drug’s action. Then they can look further upstream in the pathway to find druggable targets that produce the same therapeutic effect.”
Elucidation of COPs is one of the most pragmatic applications of the biomedical question-answering system ROBOKOP (Reasoning Over Biomedical Objects linked in Knowledge Oriented Pathways), which uses a knowledge graph structure to explore links between various biomedical data types. ROBOKOP was developed by Bizon and colleagues as part of the NIH NCATS Data Translator project.
“ROBOKOP is designed to find biological pathways for a particular drug and disease or to start with the disease and find a pathway that ends at a new drug,” said Bizon. “There are growing examples of tools based on mining of knowledge graphs in the biomedical space, many in the private sector, but ROBOKOP is one of a few fully transparent and publicly available tools that enables biomedical knowledge mining for uncovering important pathways such as those encoded by COPs.”
Integrating clinical information
Clinical observations are an important source of data necessary for elucidating COPs. ROBOKOP can be used with the Integrated Clinical and Environmental Exposures Service (ICEES), which provides open, regulatory-compliant access to clinical data—including electronic health record data—that is integrated with environmental exposures data.
“Because a lot of medical treatments are found by serendipity or through trial and error, their mechanism of action may not be known,” said RENCI collaborator Kara Fecho, who led a team that developed tools that make it possible for ROBOKOP to access this clinical data. “ICEES provides a source of clinical observations that capture when a certain drug improved a given symptom or disease, for example. ROBOKOP can then be used to fill in the missing pieces.”
The paper describes case studies in which researchers used ROBOKOP to figure out specific COPs. In one case, researchers investigated the biological mechanisms that might explain why doctors have observed that patients taking the heartburn medicine Pepcid seemed to have much milder cases of COVID-19 compared to patients not taking the medication. In another example, researchers used ROBOKOP to find COPs that explain clinical observations suggesting that the diabetes drug metformin might be able to treat certain cancers.
Once researchers make connections like these, they can design experiments to find out whether certain medications might be useful for other indications. In addition, with access to enough clinical and genetic data, it might be possible one day to use this approach to select the best therapy for an individual patient with a particular genetic makeup using clinical and genetic data specific to that patient. The researchers are also looking at how concepts similar to COPs might be employed in areas beyond drug discovery such as identifying the causes of rare diseases or explaining adverse drug outcomes.